At Science4Seniors we strive to take rigorous research published in Scientific Journals and make the core information accessible to all. If you want to support us please like and follow us on Facebook.
In recent years, the intersection of medical science and technology has unfurled fascinating possibilities, especially in diagnostics. Among the many marvels we've been introduced to, medical artificial intelligence (AI) is reshaping how we detect and diagnose a plethora of health conditions. One area that stands out significantly in this transformation is the potential of AI in the analysis of retinal images.
The Scope of Medical AI in Retinal Imaging
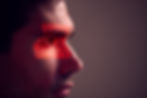
The eye, often considered the window to one’s soul, might as well be seen as a window to one’s health. The retina, in particular, is a rich tableau of veins, arteries, and nerve tissues, the health of which can often indicate not just ocular diseases, but systemic conditions as well. Recognizing abnormalities or signs of diseases in retinal images, therefore, can be pivotal in early detection and timely intervention.
Medical AI, with its pattern recognition capabilities, offers immense promise in sifting through the intricate details of retinal images. It can potentially expedite the diagnosis of eye diseases and even provide cues to systemic disorders such as diabetes, hypertension, and cardiovascular diseases.
However, there's a flip side to this promising technology. The development of effective AI models demands vast amounts of annotated data. This requirement poses two challenges:
The time-intensive nature of data annotation often slows down model development.
These models, once developed, tend to be highly task-specific and exhibit limited generalizability across varied clinical applications.
This begs the question: How can we harness the power of AI in retinal imaging while circumventing these challenges?
Enter RETFound: The Future of Retinal Image Analysis
RETFound serves as a beacon of hope in this domain. This foundation model for retinal images, unlike its predecessors, is designed to learn and understand generalizable representations from unlabelled retinal images. By focusing on self-supervised learning, it sidesteps the traditional bottlenecks of extensive data annotation, presenting a groundbreaking approach to AI-based medical diagnostics.
RETFound’s journey is a two-pronged one. Firstly, it undergoes training on an impressive 1.6 million unlabelled retinal images through self-supervised learning. This training arms the model with a foundational understanding of retinal structures and their variations. Subsequently, it is adapted to specific disease detection tasks using explicit labels.
Outperforming the Best: The Efficacy of RETFound
The brilliance of RETFound is not just in its methodology but also in its efficacy. Studies and comparisons have revealed that once adapted, RETFound consistently surpasses several contemporary models. Whether it’s the diagnosis and prognosis of sight-threatening eye diseases or the prediction of complex systemic disorders like heart failure and myocardial infarction, RETFound proves its mettle by requiring fewer labelled data.
This is particularly groundbreaking for several reasons:
Efficiency: With the ability to perform at par, if not better, with significantly less labelled data, RETFound reduces the burden on medical professionals who often need to annotate these datasets.
Broader Applications: Given its foundational nature, RETFound isn't just limited to one specific task. It offers a more versatile solution, paving the way for broader clinical AI applications, all stemming from retinal imaging.
Faster Adaptation: With the primary foundation already set, adapting RETFound for varied tasks becomes more streamlined, potentially accelerating the development of newer diagnostic tools.
Looking Ahead: The Future is Bright with RETFound RETFound is not just another AI model; it's a testament to how AI can evolve to become more inclusive, efficient, and effective in medical diagnostics. By mitigating the challenges of annotation and task-specific limitations, RETFound champions a novel approach to AI in healthcare, one that is poised to revolutionize retinal imaging diagnostics. In the grander scheme, what RETFound promises is a future where AI and healthcare walk hand in hand. It's a future where AI doesn't replace medical professionals but works alongside them, relieving them of mundane tasks, and allowing them to focus on what truly matters: patient care. In conclusion, as we stand at the cusp of technological advancements in medical diagnostics, RETFound emerges as a beacon of what the future holds. It’s not just about diagnosing eye conditions but about understanding the myriad ways our body communicates its health. The real triumph of RETFound lies not just in its technical prowess but in its potential to change lives, one retinal image at a time.
From: https://www.nature.com/articles/s41586-023-06555-x